Update on The Gartner Hype Cycle for Machine Learning
In 2017, the term "machine learning" had entered modern parlance enough for it to be included in the 2017 Gartner Hype Cycle for Emerging Technologies. Gartner doesn't include duplicate entries, year-over-year, so this is somewhat of a big deal.
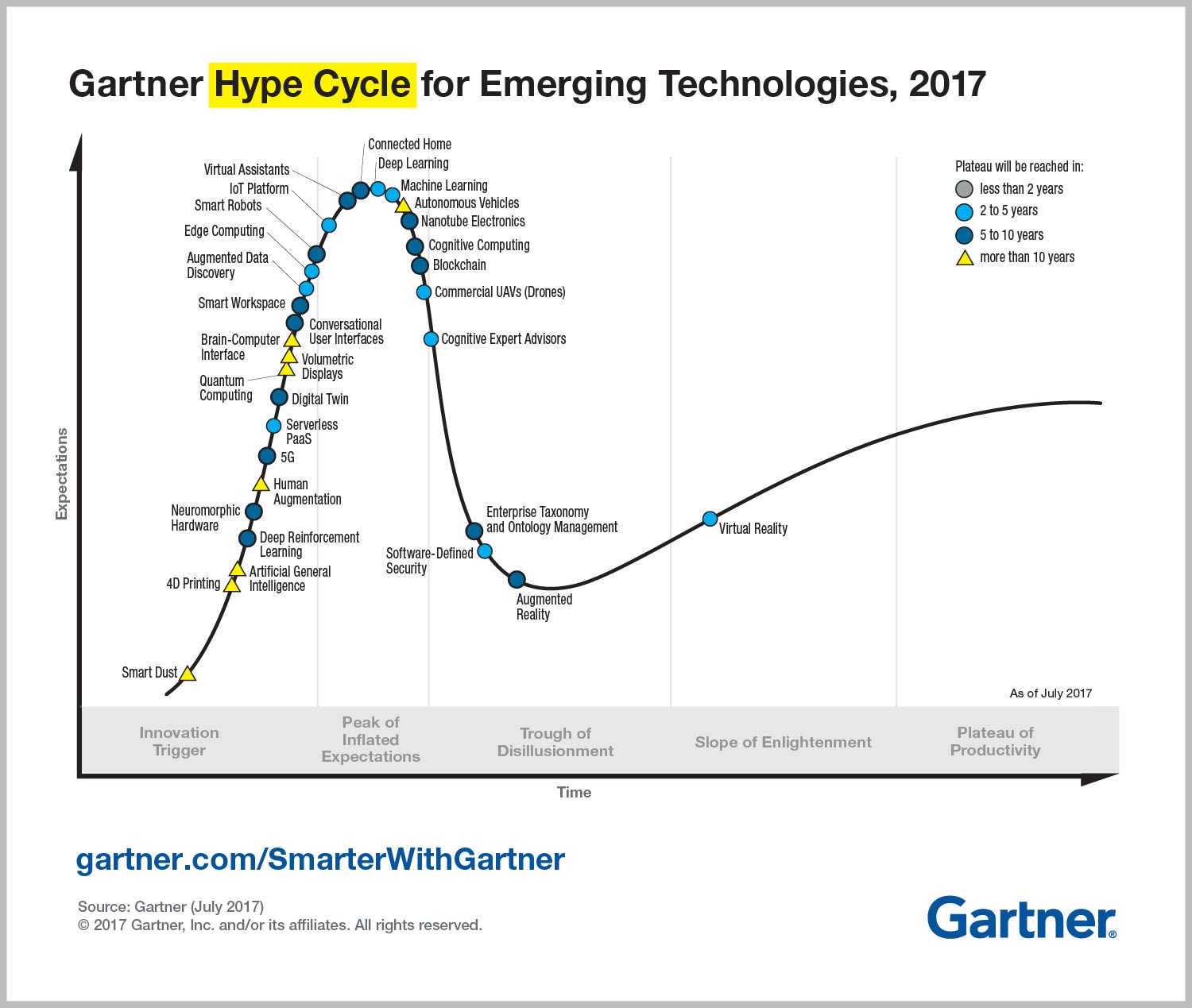
In July 2017, Gartner said that machine learning was at the "peak of inflated expectations", as in, overly hyped. But, they also said that it would reach a "plateau of productivity" in 2 to 5 years.
Based on what I hear, talking to businesses every day about machine learning solutions, I think this prediction rang true. Businesses, now more than ever, understand the relationship between statistical analysis, machine learning, and AI, and they are taking very measured and conservative steps towards implementing it in their everyday business processes.
Example: Predictive Photo Editing
One of our clients sells a photo-editing software that attempts to create perfect single-subject pictures, including background replacement, shadowing, and color correction, all from screenshots collected from a brief video recording.
In their own right, the multiple machine learning models that went into this product are impressive, but the results can't be perfect 100% of the time. The best we can hope for is that the photos are excellent 80% to 90% of the time, such that we produce a considerable time-save for businesses processing thousands of photos per day.
As a consequence, part of this software we built includes Photoshop-like manual image editing tools as fallbacks for when the automagically produced result is not perfect. Both the client and the client's customers understand that machine learning is a fuzzy process that can't be expected to produce perfect results all the time, so they happily (rather than begrudgingly) fall back on the manual editing tools when they need to.
Overall, it has created a much more productive working environment without removing the human factor from a very artistic enterprise.
Example: Investment Guidance
Another one of our clients makes a large volume of really small investments that individually come with a lot of metadata. It might seem obvious to some readers how machine learning can be applied effectively here. But, I do not think it is so obvious how to create an effective workflow here.
At the end of the day, real money and real jobs are on the line with these investments, and plausible extenuating circumstances can exist. So, how do you get machine learning involved here, at all?
Financiers are no strangers to scoring algorithms that rank investments on a scale of F to AAA, or 0 to 100. The algorithm we implemented for this client is only different in that it predicts a unique time series of cash flows on each investment. So, once they input the potential investments into the system, they can model the approximate outcome of their entire portfolio, while still maintaining the flexibility to make exceptions and intelligently navigate client relationships.
The Peak of Inflated Expectations
Data scientists in our industry often joke that their entire job consists of ...
- Importing a machine learning package
- Inserting the data
- Shipping the results
... which, in the minimal case, is all of 10 lines of code. This joke references what was once the Peak of Inflated Expectations in the profession -- that machine learning was a free plug-and-play process.
The Plateau of Productivity
At Conlan Scientific, we understand that effectively applying machine learning is as much of a process engineering problem as it is a data engineering problem. As time goes on, more businesses start to understand and appreciate this concept, which symbolizes the Plateau of Productivity -- when businesses can assess machine learning as one of many potentially viable sources of innovation.